ABOUT THIS COURSE
This unique leadership course demystifies AI for leaders. It introduces the broad spectrum of AI technologies and applications, then drills into the most common and practical areas that drive substantive transformation when properly applied.
This short course addresses the cultural, environmental, team and resource issues that directly impacts AI adoption in medium and large organizations. It is designed and facilitated by active and highly seasoned strategic consultants who frequently encounter the vast challenges and realities of modern organizational AI and machine learning implementation.
Leaders will return with a new mindset and powerful steps toward forming a coordinated AI-driven practice that generates perpetual benefit. While others remain fascinated by technology and hype, this benefit will deliver unfair advantage and establish your organization as an AI-driven leader.
YOUR TEAM WILL LEARN HOW TO
-
- Advance top leadership’s literacy and attitude toward AI, machine learning and analytics
- Identify and qualify opportunities that align with organizational priorities
- Manage a portfolio of AI programs and projects that drive measurable benefit
- Assign, inspire and retain the various roles and functions of the analytics team
- Convey a formal framework for establishing a comprehensive machine learning practice
- Form the foundation required to build a federated AI and analytics competency for scalable affect
GEARED TO
-
- C-Suite Executives including CEOs, CIOs, CAOs, CTOs, Board and Steering Committee Members
- Line of Business General Managers responsible for driving measurable value from analytics
- Organizational Leaders that are accountable for advancing analytics maturity and competency
- Technology Planners who prioritize corporate investment
- Consultants desiring to shift their clients’ fascination with technology to a focus on a design strategy for driving measurable and residual value
TOPIC OUTLINE
SESSION I – STRATEGIC ORIENTATION TO AI
- Introduction to AI, machine learning and organizational analytics
- Trends within the AI-competitive organization
- The power of classic ‘boring’ AI
- Prioritization of Generative AI, ChatGPT and other LLMs
- Analytic opportunity identification, qualification and prioritization
- Organizational resistance and developing a culture for change
- Staging the organizational mind shift to data-driven decisioning
- Attracting and hiring the right talent
- The roles and functions of the fully-formed AI team
- Specialization in analytic project teams
- Motivating adoption by domain experts, end users and leadership
- Monitoring and advancing organizational AI performance
- “Democratizing” analytics: Advantages and risks of “self-service”
- Common strategic failures
- Portfolio -> Program -> Project
- Rush to Implementation
- Too Many Proofs of Concept
- Before you begin: Start with Phase Zero
SESSION II – HOW TO THINK LIKE A DATA SCIENTIST
- Stats 101 in ten minutes
- A / B testing and experiments
- BI vs predictive analytics
- IT’s role in predictive analytics
- Statistics and machine learning: complementary or competitive?
- Primary project types
- Predicting a value given specific conditions
- Identifying a category given specific conditions
- Predicting the next step in a sequence
- Identifying groups
- Common analytic algorithms
- Regression
- Decision Trees
- Neural Networks
- Genetic Algorithms
- Ensemble Modeling
- Popular tools to manage large-scale analytics complexity
- R and Python
- Hadoop, MapReduce and Spark
- Data Mining “workbenches”
- Performing a data reconnaissance
- Building the analytic sandbox
- Preparing train / test / validation data
- Defining data sufficiency and scope
SESSION III – THE CAO’s ROADMAP
- The Modeling Practice Framework™
- The elements of an organizational analytics assessment
- Project Definition: the blueprint for prescriptive analytics
- The critical combination: predictive insights & strategy
- Establishing a supportive culture for goal-driven analytics
- Defining performance metrics to evaluate the decision process
- What is the behavior that impacts performance?
- Do resources support stated objectives?
- Leverage what you already have
- Developing and approving the Modeling Plan
- Selecting the most strategic option
- Planning for deployment
- What will the operational environment be?
- Who or what is the end consumer?
- How do results need to be purposed or presented?
- Measuring finalist models against established benchmarks
- Preparing a final Rollout Plan
- Monitoring model performance for residual benefit
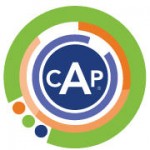